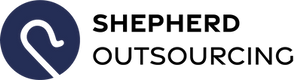
Shepherd Outsourcing opened its doors in 2021, and has been providing great services to the ARM industry ever since.
About
Address
©2024 by Shepherd Outsourcing.
Data analytics is changing the game in debt collection, and it’s not just a buzzword. Research suggests that agencies utilizing AI for predictive analytics have reported a 25% increase in recovery rates. If you’re in the business of recovering payments, understanding your data is no longer optional. Imagine having the power to predict which customers will likely default on payments before they even miss a due date. That’s exactly what data analytics brings to debt collection. You need real insights and actionable data that help you optimize recovery rates, reduce risks, and improve customer relationships.
With data analytics, you can analyze payment behaviors, recognize trends, and make sound decisions rather than chasing debts blindly. From predictive modeling to real-time monitoring, analytics helps you focus on accounts that matter most, increasing efficiency and success rates.
But how does it all work, and why is it becoming a game-changer in debt collection? Let’s break it down and explore how leveraging data can transform your approach, making debt recovery smarter and more effective than ever.
Data analytics in debt collection is all about using facts and figures to guide your decisions. Rather than depending on speculation or outdated methods, you’re using real-time insights to shape your strategy. It includes collecting, structuring, and examining data from different sources, like payment history, customer behavior, credit scores, and communication patterns. In simple terms, debt collection analytics turns raw data into useful insights. It helps you answer key questions like:
By using analytics, you’re not just managing debt but strategically controlling it.
So, why does this matter to you?
Data analytics is central to modern debt collection, which turns raw information into powerful strategies. It helps you go beyond just tracking overdue payments—you’re predicting outcomes, optimizing resources, and improving customer interactions.
Here’s how data analytics shapes the debt collection process:
While analytics plays a crucial role in streamlining and optimizing debt collection, it's also important to consider the human side of the process. Sometimes, debtors need personalized assistance to navigate their financial challenges. This is where Shepherd Outsourcing comes in.
Shepherd Outsourcing offers a comprehensive debt settlement service as an intermediary between debtors and creditors. Talk to us for more information.
Debt collection analytics follows a structured approach to improve recovery rates while maintaining a customer-centric approach. Here are the key stages:
At this stage, data analytics helps you spot customers likely to default. You can identify accounts that need early intervention by analyzing payment history, spending patterns, and credit scores. Proactive engagement, such as gentle reminders or flexible payment options, can prevent overdue accounts from escalating.
Once high-risk accounts are identified, analytics segment them based on factors like debt amount, repayment history, and communication responsiveness. Risk scoring assigns priority levels, ensuring collection teams focus on cases that require immediate attention while offering alternative solutions to others.
For customers struggling to pay, analytics-driven insights help tailor hardship plans, such as revised payment schedules or reduced interest rates. This approach balances recovery efforts with customer well-being, increasing the chances of successful debt resolution.
By leveraging data at each stage, you can streamline debt collection while maintaining positive customer relationships.
Debt collection analytics relies on several key components to improve recovery rates, optimize collection strategies, and enhance customer interactions. Here are the essential elements:
The foundation of debt collection analytics is collecting and collecting data from various sources, such as payment history, credit reports, customer interactions, and financial statements. This unified data approach ensures that collection teams have a complete view of each debtor’s financial behavior, allowing for informed decision-making and targeted collection efforts.
Not all debtors are the same, so segmentation plays a crucial role. Businesses can prioritize accounts by categorizing customers based on payment behavior, debt amount, financial stability, and responsiveness. High-risk customers may require immediate attention, while low-risk customers might just need a simple reminder to make timely payments.
By utilizing historical data and AI-powered models, predictive analytics helps anticipate which customers are most likely to default or settle their debts. Risk scoring provides each debtor with a numerical value, allowing collection teams to focus on accounts requiring proactive intervention rather than a one-size-fits-all approach.
Transparent communication helps in debt recovery. Debt collection analytics determine the most suitable communication channels—phone calls, emails, or SMS—and the best timing for contacting debtors. Personalized messaging and automated follow-ups enhance engagement, making it easier to encourage timely repayments.
Understanding how customers make payments and their preferred methods allows businesses to design more effective collection strategies. Some debtors may require flexible payment plans or incentives to settle their dues. By analyzing trends in late payments, partial payments, or missed deadlines, businesses can tailor their approach to improve repayment success.
Adhering to legal and ethical standards in debt collection is critical. Analytics helps ensure that all collection activities comply with financial regulations, protecting businesses from legal risks. It also promotes fair treatment of customers, reducing the chances of disputes and negative customer experiences.
The effectiveness of debt collection efforts must be continuously monitored. Analytics enables businesses to monitor the effectiveness of different collection strategies, agent performance, and debtor response rates. These insights help refine collection tactics, optimize resource allocation, and improve efficiency in recovering outstanding debts.
Also Read: Understanding Debt Restructuring: Process, Types & Key Benefits
The debt collection industry is experiencing major growths, fueled by technological innovations and shifting consumer expectations. A study indicates that the debt collection software market is projected to grow to USD 7.96 billion by 2030.
Staying abreast of these changes is crucial for organizations aiming to enhance recovery rates and maintain positive customer relationships. Here are some key trends shaping the future of debt collection analytics:
While data analytics can dramatically improve debt collection processes, it comes with its own set of challenges. Here’s a look at some common hurdles and how to address them:
Incorporating data analytics into debt collection processes is important for businesses striving to improve efficiency, reduce risks, and maximize recovery rates. By leveraging data to identify patterns, predict behavior, and personalize communication strategies, companies can achieve better outcomes with less effort. However, it’s important to remember that successful debt collection isn’t just about data—it’s also about providing tailored support and solutions for debtors.
That’s where Shepherd Outsourcing comes in. As experts in debt management, we specialize in negotiating favorable settlement terms and offering personalized debt plans. Our focus on legal compliance and financial counseling ensures businesses and debtors benefit from a smoother, more effective collection process.
1. How does data analytics improve the debt collection process?
A: Data analytics helps streamline debt collection by identifying high-risk accounts, predicting repayment likelihood, and segmenting customers based on payment behavior. It enables businesses to create targeted collection strategies, automate communication, and optimize recovery rates while maintaining a customer-friendly approach.
2. What types of data are used in debt collection analytics?
A: Debt collection analytics relies on data such as payment history, credit scores, customer interactions, financial stability, and behavioral patterns. This information is collected from various sources, including banking records, past due accounts, and communication logs, to provide a comprehensive view of each debtor.
3. How does predictive analytics help in debt recovery?
A: Predictive analytics uses historical data and AI models to assess the probability of repayment or default. By assigning risk scores, businesses can prioritize collection efforts on accounts that need immediate attention while offering flexible repayment solutions to those willing but struggling to pay.
4. Can data analytics improve customer relationships in debt collection?
A: Yes, analytics-driven debt collection is more strategic and less aggressive. By understanding a customer’s financial situation and payment behavior, businesses can offer personalized repayment options, improving trust and reducing disputes. This approach fosters long-term customer relationships while increasing recovery rates.
5. What role does automation play in debt collection analytics?
A: Automation enhances efficiency by sending timely reminders, scheduling follow-ups, and selecting the best communication channels based on debtor preferences. It reduces manual effort, speeds up the collection process, and ensures consistent, professional, and data-driven interactions with customers.